Neural Enhanced Belief Propagation for Multiobject Tracking
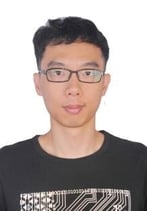
About this Topic:
Multiobject tracking (MOT) is a key challenge in a wide range of applications, such as autonomous navigation and applied ocean sciences. MOT is complicated by object appearance and disappearance, data association ambiguities, and occlusion. Conventional Bayesian methods for MOT, e.g., methods based on belief propagation (BP), entirely rely on a statistical model. This fully model-based approach can lead to highly suboptimal estimates when there is a mismatch between the statistical model and the true data-generating process. In this webinar, the presenter will introduce a model-based and data-driven MOT method that relies on neural-enhanced belief propagation (NEBP) to integrate hand-designed statistical models with neural networks. In this way, the proposed hybrid MOT approach can combine the advantages of model-based and data-driven estimation, i.e., it makes use of information learned from raw sensor data but also relies on a scalable statistical model. NEBP for MOT is currently leading the nuScenes tracking challenge for LiDAR data.
About the Presenter:
Mingchao Liang received the B.Eng. degree in electronic engineering from the Chinese University of Hong Kong, Hong Kong SAR, China, in 2018. He is currently working toward a Ph.D. degree in electrical and computer engineering with Prof. Florian Meyer at the University of California San Diego, La Jolla, CA, USA.
His research interests include localization and tracking algorithms, graphical models, machine learning, and deep learning.
Mr. Liang was the recipient of a Qualcomm Innovation Fellowship in 2022. He serves as a reviewer for the IEEE Transactions on Aerospace and Electronic Systems, the IEEE Transactions on Wireless Communication, and the IEEE Transactions on Signal Processing.
Want to learn more about upcoming events & webinars?
Visit the events section of the Signal Processing website to see all upcoming lectures, workshops, webinars, and more.